Generate accurate, realistic synthetic data with an unmatched privacy guarantee
Patterns and outliers in synthetic data can reveal sensitive information. Differential privacy preserves data utility while preventing re-identification.
Perspective
To provide robust privacy protection, including against rapid developments in privacy attacks, synthetic data should be generated using differentially private algorithms.”
Key uses
Train ML/AI models without
exposing sensitive information
Differential privacy allows machine learning and AI models to be trained on synthetic data without exposing sensitive information from the training dataset.
Unlock and increase
internal data sharing
DP enables organizations to share synthetic data internally without risking exposure of sensitive information from the original dataset.
Retain data, safely,
legally and forever
DP allows organizations to retain synthetic data indefinitely while complying with U.S. and GDPR regulations that limit the retention of personal data.
Monetize data
sets, safely
Differentially-private synthetic data sets can be sold to researchers and other enterprises, offered through data marketplaces, and used to enhance existing data products.
Perspective
about
How can differential privacy assure data fidelity and accuracy?
To achieve high accuracy while offering the robust guarantees of differential privacy, generation techniques need to carefully optimize for the preservation of statistical properties in the source data.
Leveraging their award-winning algorithms, which won NIST’s Differential Privacy Synthetic Data Competition, Tumult Labs' approach ensures synthetic data retains crucial insights with high accuracy.
How differential privacy protects AI/ML workflows
Differentially-private synthetic data resists
attacks and assures privacy in AI/ML training
Input to predictive ML is sensitive
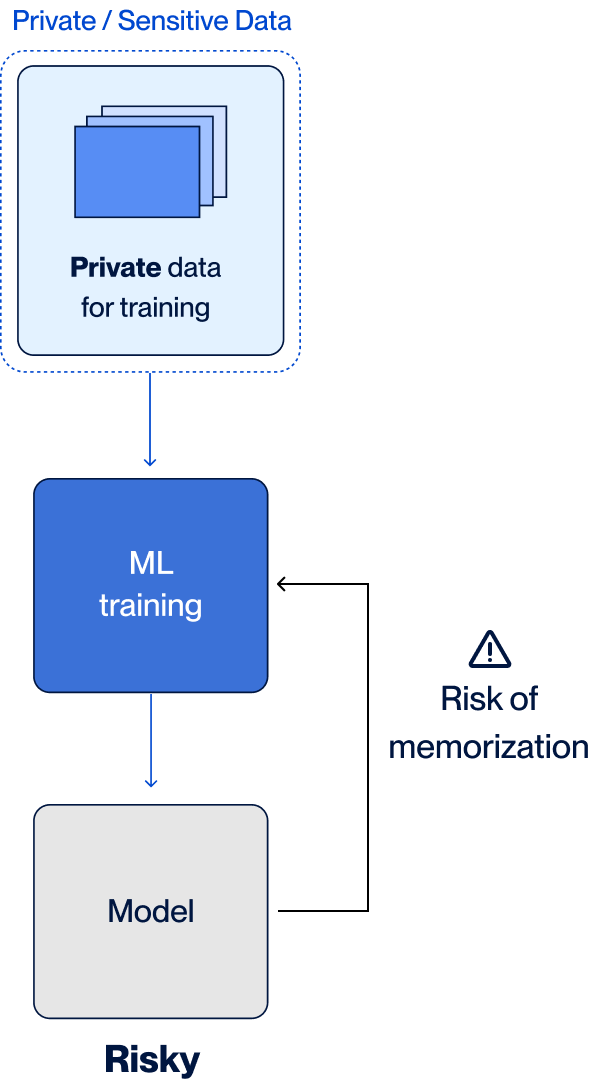
Differentially-private synthetic data replaces sensitive training data
